Harnessing the Power of Annotation Tool Machine Learning for Businesses
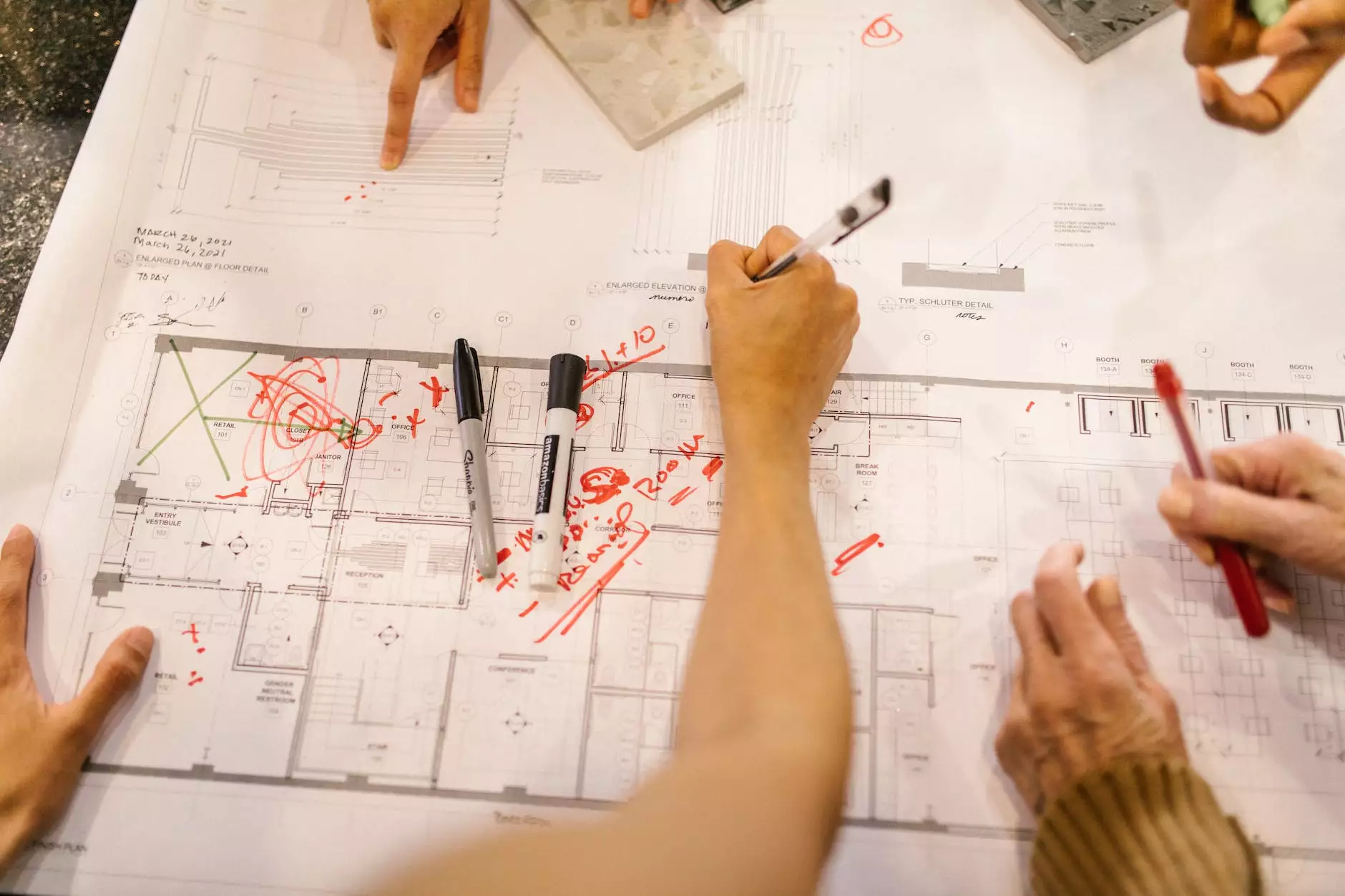
In the rapidly evolving digital landscape, businesses are increasingly turning to innovative tools to streamline operations and enhance service delivery. One such transformative tool is the annotation tool machine learning. Integrating this technology into business processes can lead to significant improvements in efficiency, accuracy, and customer satisfaction. In this article, we will delve deeply into the various facets of this technology, exploring its applications, benefits, and how it can revolutionize the home services and locksmith industries.
Understanding Annotation Tool Machine Learning
Before we explore the benefits and applications of annotation tools, it's essential to understand what machine learning annotations are. At its core, machine learning involves training algorithms to recognize patterns in data. Annotation tools assist in this process by labeling data, which provides the necessary context for machines to learn from it. In practical terms, this means taking raw data—be it images, text, or videos—and annotating it so that machine learning models can be trained to make predictions or classifications.
The Basics of Data Annotation
Data annotation can be conceptualized as a two-step process:
- Data Collection: Gathering large sets of raw data relevant to a specific task.
- Data Labeling: Annotating or labeling this data to provide context, enabling machine learning algorithms to understand and learn from it.
For instance, if a business is utilizing machine learning for image recognition, it must first collect images and then annotate these images with labels (like “house,” “car,” or “person”) so that the algorithm can learn to identify these objects accurately in new images.
Why is Annotation Important for Machine Learning?
Accuracy and effectiveness of machine learning models are heavily dependent on the quality of the training data. Properly annotated data not only improves the performance of the model but also reduces biases that can arise from unannotated or poorly annotated datasets. This is particularly crucial in industries where precision is paramount, such as in home services and locksmiths.
Applications of Annotation Tool Machine Learning in Business
Annotation tool machine learning is applicable in various sectors, including:
- Customer Service: Chatbots powered by machine learning can understand and respond to customer inquiries more effectively by analyzing past interactions.
- Image Recognition: Home services can utilize machine learning to better analyze properties, whether for maintenance scheduling or repair assessment.
- Predictive Maintenance: By analyzing customer service requests and property data, businesses can predict when maintenance will be needed, thus improving service delivery.
- Locksmith Services: Automating the identification of service calls based on patterns in data can enhance response times and overall efficiency.
The Benefits of Utilizing Annotation Tool Machine Learning
The incorporation of annotation tool machine learning brings numerous benefits:
1. Enhanced Efficiency
Automation reduces the time employees spend on mundane tasks. Instead, they can focus on more critical areas within the business, substantially improving overall productivity.
2. Improved Accuracy
Systems that have been properly trained using annotated data tend to be more reliable, resulting in fewer errors in service delivery. This is vital for maintaining trust and credibility within customer interactions.
3. Increased Scalability
As businesses grow, the volume of data increases. Annotation tools can help scale data management systems efficiently, accommodating larger datasets seamlessly without sacrificing performance.
4. Better Decision-Making
Rich data insights derived from well-annotated datasets empower business leaders to make informed decisions based on actual usage patterns and predictions.
How to Implement Annotation Tool Machine Learning in Your Business
Implementing an annotation tool involves several steps:
- Define Your Objectives: What do you aim to achieve with machine learning? Identify key performance indicators (KPIs) relevant to your business.
- Choose the Right Tools: Research and select annotation tools that best suit your needs, such as Labelbox, SuperAnnotate, or VGG Image Annotator.
- Train Your Team: Ensure that your team understands how to utilize these tools effectively for maximum productivity.
- Start Annotating: Begin the annotation process on select datasets, gradually expanding as your team gains experience.
- Evaluate and Optimize: Regularly review the performance of your models and the quality of your annotations to implement improvements.
Real-World Examples of Annotation Tool Machine Learning Applications
Case Study: Home Services Industry
A leading home services provider implemented an annotation tool to improve its image recognition capabilities. By annotating service request images with labels indicating specific issues (leaks, electrical faults, etc.), the company trained its machine learning model to prioritize urgent requests, leading to a 30% reduction in response time and significantly higher customer satisfaction scores.
Case Study: Locksmith Services
A locksmith service provider utilized machine learning to analyze historical customer calls and successful resolutions. By annotating call transcripts with relevant insights (for instance, common lock types and the frequency of certain problems), they were able to automate dispatch decisions, ensuring that the right technician was sent for each job, resulting in faster service delivery and improved customer feedback.
The Future of Annotation Tool Machine Learning
As technology continues to advance, the potential for annotation tool machine learning to drive further innovations in business operations is vast. With improvements in AI and machine learning algorithms, businesses can look forward to more sophisticated models that require less manual annotation and can adapt more swiftly to changing market conditions.
Key Trends to Watch
- Automated Annotation: Emerging tools that leverage AI to automate aspects of the annotation process will decrease the burden on human annotators.
- Real-Time Learning: Models that can learn and adapt in real-time based on incoming data will increase responsiveness and adaptability.
- Focus on Ethics and Bias Mitigation: As machine learning models become integrated into societal functions, addressing ethical issues and bias in data will gain importance.
Conclusion
Incorporating an annotation tool machine learning strategy can unlock significant advantages for businesses, especially for those in home services and locksmiths. By enhancing operational efficiency, accuracy, and scalability, organizations can provide better services, improve customer satisfaction, and stay ahead of the competition. As we venture further into the future, embracing these technologies will be vital for businesses aiming to thrive in an increasingly data-driven world.
For more insights on how to integrate these tools into your operational framework, visit keymakr.com and explore various resources that can guide you in harnessing the power of machine learning effectively.